We live in a data-driven world. According to Statista, the total amount of data created, captured, copied, and consumed globally is forecast to reach more than double in 2025 compared to 2021. Much of this data is personal or sensitive, representing a threat to our privacy if it is leaked and costing millions to companies when accidentally there is a data breach.
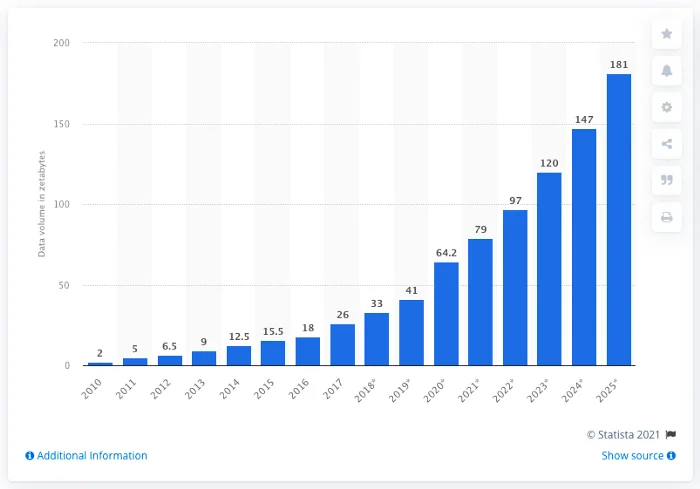
Also, Artificial Intelligence (AI) solutions need tons of data to be created. To make forecasts, avoid fraud or just understand their customers better, companies need to analyze those lakes of data. But one thing is what you want and another totally different thing is what you can do. Privacy is too important to all, and for that reason, regulations are everywhere. One good example in Europe is the General Data Protection Regulation (GDPR for short).
But this won’t stop here, according to Gartner, 65% of the world’s population will have it’s personal data covered under modern privacy regulations. Then the engineers, data scientists, analysts and the rest of AI-alike professionals formulae some questions: how we feed the Machine Learning (ML) models, how we improve the current algorithms or how we develop that application if we can’t access the real data. The answer for all of them has been there for a while, but it wasn’t good enough until the advancements made in Deep Learning (DL) during the 10s of the 21st century. Today, we can say it is the definitive solution: Synthetic Data.
Oh how I didn’t think that… wait… what do you mean by Synthetic Data?
Even when the Synthetic Data works also with images and text, let’s explain it for the case of structured data, the one you find on tables, with rows and columns.
Imagine the data is milk, the people are the cows and you want to add milk to your breakfast coffee (apply that unsupervised ML algorithm), but you are vegan (this is the regulation — GDPR). Then the Synthetic Data will be the soy milk. It is similar to the original and it keeps the value being totally useful for the same purpose. Then you have protected the subjects’ (cows) “privacy” because it is impossible to re-identify them looking at the soy-based subjects and you have solved your problem.
That’s why the Synthetic Data Generators are used to anonymize data.
But… Why is it so important?
It is extremely important because it opens the door to an ocean of possibilities. Having Synthetic Data that keeps the information but is safe, because it “anonymizes” the original data surpassing other data anonymization techniques that have proven to have flaws, allows companies to use and share data that was banned before. This will result in new or improved apps, products, ML models, analysis, better understanding, cross-industry results, etc. The sky will be the limit for innovation, accessing data that was denied before.
Companies will be able to extract value and monetize data, raising the bar. Sometimes we hear the term Synthetic Data Revolution, and can’t be more accurate. Having access to Synthetic Data will change the world the same way ML and DL did.
More on the data science side, it will also have an impact on AI & ML models. The Synthetic Data Generators allows, based on the amount of data used to create the generator model, to create all the data you want. This will lead, in some cases, to increased performance for the current ML models in the market. Citing Garter one more time, they estimate that by 2022, 40% of AI & ML models will be trained on Synthetic Data.
Be ready, the revolution is already here!
References
1. Volume of data/information created, captured, copied, and consumed worldwide from 2010 to 2025. Arne Holst, Jun 7, 2021.
2. Gartner Says By 2023, 65% of the World’s Population Will Have Its Personal Data Covered Under Modern Privacy Regulations. Gartner, September 14, 2020.
3. Maverick Research: Use Simulations to Give Machines Imagination. Anthony Mullen, Magnus Revang, October 8, 2018.